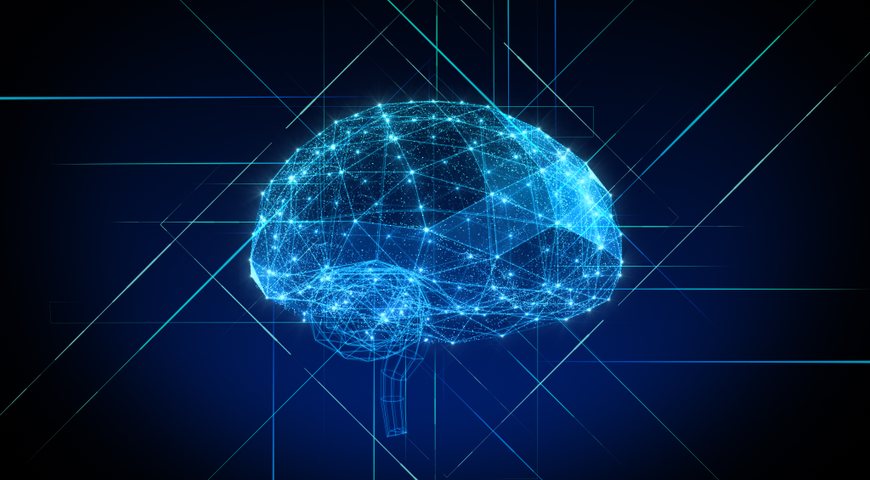
The last years have seen the indisputable rise of artificial intelligence as the one differentiator of a truly cutting-edge business. Businesses that want to stay competitive do AI.
Yet, a 2018 research by Gartner which still unfortunately holds true reports that a staggering 85% of Machine Learning projects fail before even going to production. Is AI not worth the hype? On the contrary!
A lack of a cohesive AI strategy, misapplications of AI to business use cases and poor organizational alignment are the real culprit of these failures. AI has shown beyond a doubt the potential to solve real business and world problems while unlocking millions worth of savings and revenue, that is when businesses don’t feed the hype and correctly look at AI as an engineering discipline.
This review provides the reader with the baseline knowledge needed to understand what AI can really do for businesses by outlining an easy-to-digest overview of what artificial intelligence is, with a walk-through of the most relevant terminology, approaches and good vs bad real-world applications.
The terminology of AI
Artificial intelligence, machine learning, deep learning and machine intelligence are terms often used interchangeably to refer to smart automated applications, but they do have different meanings — and understanding their differences is the first step towards a successful AI adoption.
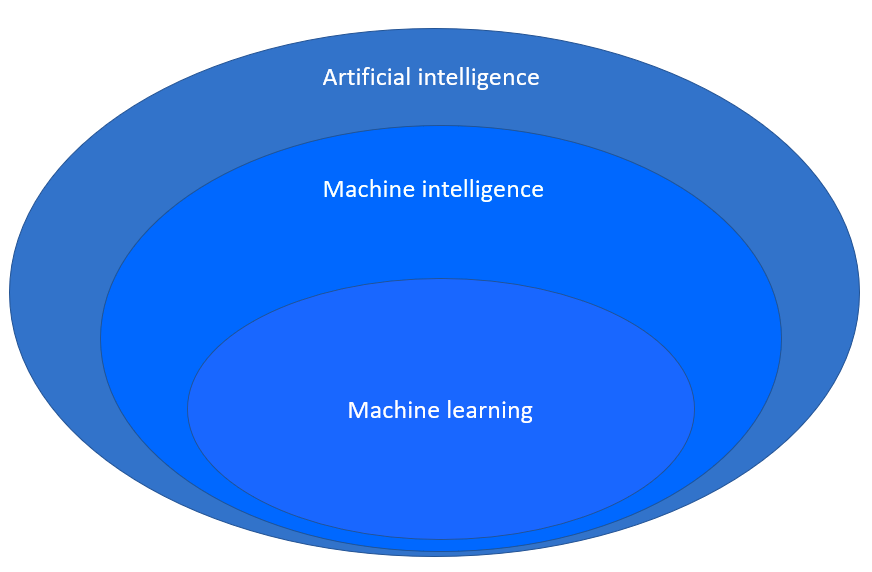
Artificial Intelligence is the discipline that aims to create human-like machine intelligence. Different mathematical and logical approaches can be used to achieve this smart behavior.
Machine learning is the specific subfield of AI which uses mathematical models of data to provide predictive power to computer systems. When these mathematical models take the shape of neural networks, then we’re talking about deep learning.
Finally, when multiple inputs and multiple ML models are used to create complex systems, additional automation and prioritization processes are introduced which belong to a rising discipline called machine intelligence.
These various implementations of AI can mimic human intelligence with varying levels of specialization. Depending of the number of tasks that the AI system has learnt, the following three parallel subsets can be derived:
- Weak, or narrow AI: the machine can intelligently perform one task only.
- General AI: the machine can intelligently perform a few tasks, but is not a cognitive entity.
- Strong, or super AI: the machine can continuously learn and self-define new tasks, while demonstrating a sense of self awareness.
Narrow AI is what real-world AI applications achieve. Through the use of advanced ML techniques on small and big data, machines have proven that they can successfully learn high levels of specialized knowledge in a variety of single domains — from risk intelligence to personalized customer experience, and often outperform human capabilities.
Beyond this, Machine Intelligence holds the potential to be the step towards general and strong AI, as it focuses on solving complex problems which not only involve learning but also problem solving and prioritization.
The approaches behind AI
One famous saying is often cited by ML practitioners, which goes “garbage in, garbage out.”
Before looking at the approaches behind AI, it is fundamental to understand that data drives AI applications. The right data needs to be provided as input to the AI models during training for them to derive useful outputs.
Data can be classified in the following three categories depending on its format:
- Structured data. This is tabular data, where information is structured in rows and columns within a predefined schema. This data is stored in databases, and it is the easiest to search and organize.
- Unstructured data. This is data that cannot be stored in a table, such as documents and media (audio, images and video). This data is stored in data lakes, NoSQL databases and blob storages.
- Semi-structured data. This is data that comes with some consistent characteristics but not a rigid structure, such as logs, xml and other markup languages, emails.
More than 80% of data in the enterprise is unstructured data, which is also why data engineering is often cited as a must-have skill for a ML team.
Through data preprocessing and feature engineering, data sources of all formats can be reshaped in useful representations for ML model training. Below, the most common modeling approaches are presented for real-world applications in AI and its main subfields, with a final highlight of when they are not best applied too.
● Modeling for AI
The two most common approaches for pure AI are:
○ Pathfinding, which aims to provide the optimal route between two or more points. Implementations are variations on Dijkstra’s algorithm for shortest path finding on a weighted graph.
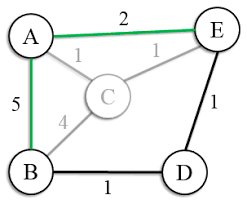
These models can be used for GPS, or to optimize a delivery service or a rail network.
○ Symbolic logic, which represents human knowledge in a declarative form by means of facts and rules defined as logical assertions.
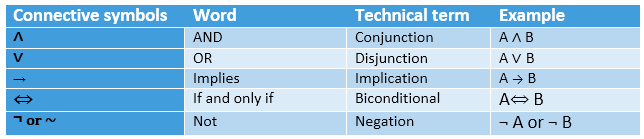
Expert knowledge needs to be available and encodable, which is uncommon for real-world applications with the exception of games such as chess or specific expert systems in the legal and medical fields.
Applications that require expert knowledge or small data are often best solved with pure AI. When data is available, it is not recommended to use ML approaches over pure AI approaches even when the latter are feasible (e.g., chatbots, gaming, etc.).
● Modeling for Machine Learning
Most real-world applications use Machine Learning. ML approaches can be grouped in the following categories:
○ Supervised learning, where the output (“label”) that the model is trying to learn is known.
The model can perform a classification task, where the label is a specific category such as cat / dog or churn / not-churn, or a regression task, where the model learns to predict a numerical value such as sales revenue or customer lifetime value.
o Unsupervised learning, where data is unlabeled.
The model can cluster the unlabeled data into groups by maximizing within-group similarity and minimizing inter-group similarity, or perform dimensionality reduction to reduce the number of data inputs to a lower size while keeping most of its information.
○ Semi-supervised learning, where a small subset of data is labeled while the rest is unlabeled.
This learning scenario is most typical of Deep Learning applications, where models such as Generative Adversarial Networks are powerful enough to learn from the labeled data and generalize to the unlabeled set. The most typical application is medical imagery, such as CT scans or MRIs, where labeling data is a time- and cost-intensive task for experts only.
○ Reinforcement learning, where an environment is created for an AI agent to learn within.
The agent attempts to find the optimal way to accomplish a task by repeatedly simulating attempting the task and optimizing for a reward. Typical applications are self-driving cars, gaming and industry automation.
Most narrow AI applications on structured data such as customer churn, product recommendations, or email marketing, are best solved with pure ML approaches. For more complex systems where multiple inputs and models are required, it is recommended to explore Machine Intelligence solutions.
It is also important to note that ML is not always the answer. Sometimes simple heuristics or rule-based engines behave as good as ML applications, e.g., when data dimensionality is small or data quality is not good or the task has a small number of degrees of complexity, while providing the benefit of minimizing engineering requirements.
● Modeling for Deep Learning
Deep Learning is large neural networks. A neural network is composed of an input layer of the same dimension as the data, an output layer of the same dimension as the labels and 1+ layers which perform parametrized mathematical transformations that learn how to map the input data to the output labels.
Neural networks can learn how to predict an output, embed data or generate new data.
Most if not all applications based on unstructured data use DL, such as pose estimation from videos or anomaly detection from network data. Else, for applications where big data is not required, DL is often not the best solution as it is complex, not easily explainable and compute-heavy.
● Modeling for Machine Intelligence
Machine Intelligence has no default approach. It can be envisioned as a consecutive or parallel ensemble of models and objectives which allow to achieve an optimal outcome given a combination of inputs. Given the complexity of the approach, which often focuses on systems where even human performance is simply not enough, it is recommended to look at specialized third-party solutions rather than ad-hoc approaches.
Some good applications of MI are adaptive learning, where teaching is customized to each student’s performance, or supply chain management.
The most advanced application for impact and maturity is Risk Intelligence, which refers to the ability of an organization to gather information that will successfully identify uncertainties in a workplace (fraud detection, email phishing and spamming, network protection, etc.).
A MI solution provides a reliable system with automated, privacy-compliant and agreed-upon decision processes which allow businesses to respond faster with built-in severity levels that can determine the criticality of an event and enact smart response plans.
What businesses can achieve with AI
While AI aims to enable computers and machines to function with human intelligence, its goal is not to replace humans but to provide a supporting tool to automate mundane tasks and allow people to take on elevated responsibilities.
The predictions and decisions of AI models should not be taken as ground truth, but as smart suggestions to validate and act upon. The end-to-end design and automation of these systems is what often provides the most value.
In the realm of narrow AI, most business needs have been solved with Machine Learning (e.g., product identification, recommender systems, fraud detection, etc.) and the success of an AI initiative is mainly related to strategy and decision making.
With more complex applications involving big data and real-time requirements, AI can provide volume, velocity, variety, veracity and value beyond human skills, and thus be of the most impact. With the right AI solution and strategy, businesses have the chance to focus on proactive rather than reactive actions, and tap in the real hype behind AI.
Acronis Cyber Protect Cloud unites backup and next-generation, AI-based anti-malware, antivirus, and endpoint protection management in one solution. Integration and automation provide unmatched ease for service providers — reducing complexity while increasing productivity and decreasing operating costs. Try it today!
About Acronis
A Swiss company founded in Singapore in 2003, Acronis has 15 offices worldwide and employees in 50+ countries. Acronis Cyber Protect Cloud is available in 26 languages in 150 countries and is used by over 21,000 service providers to protect over 750,000 businesses.